
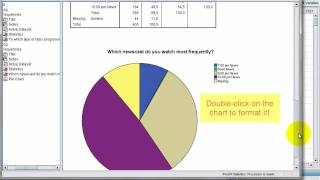
In a factorial design, there are two or more factors with multiple levels that are crossed, e.g., two dose levels of drug A and two levels of drug B can be crossed to yield a total of four treatment combinations. A factor is a variable that is controlled and varied during the course of an experiment. To understand the effect of two or more independent variables upon a single dependent variable, completely randomized factorial design is often used in medical experiments or clinical trials. The difference between high-dose group and placebo group is still statistically significant ( p < 0.001). The results of multiple comparisons show that the difference between low-dose group and placebo group is not statistically significant ( p = 0.069), which is completely contrary to the results of the independent sample t-test (Table 3). The results indicate that there is a statistically significant difference between groups as determined by one-way ANOVA ( F = 24.728, p < 0.001). Īccording to the study design, selecting “Analyze➔Compare Means➔One-Way ANOVA…” and ticking “Dunnett” in the “Post Hoc Multiple Comparisons” dialog box in SPSS, we perform one-way ANOVA and Dunnett’s post hoc test to compare each dose group with the placebo group. Thus, it is not appropriate to perform the independent sample t-test directly for comparisons with control group. These data are typical quantitative data of multigroup independent sample design, also known as the single factor design with multiple levels, and the number of levels is 3. The statistic results are presented in Table 3 (see Additional file 3 for the original data). The independent sample t-test is adopted to compare the low-dose group with the placebo group and the high-dose group with the placebo group, respectively.
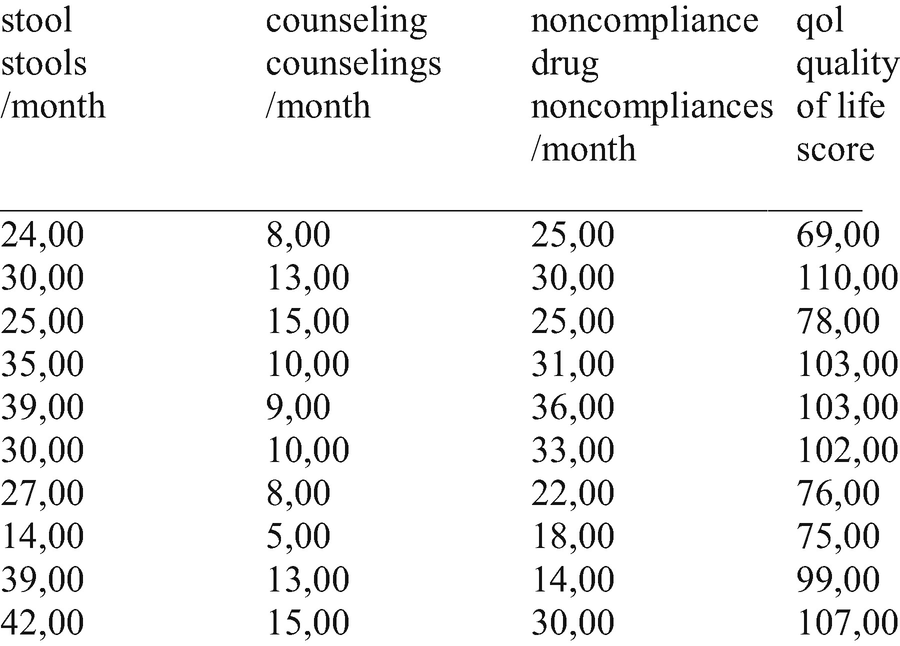
Example 3įor a new antihypertensive drug, we hope to compare the antihypertensive effect of high- and low-dose groups with that of placebo group.
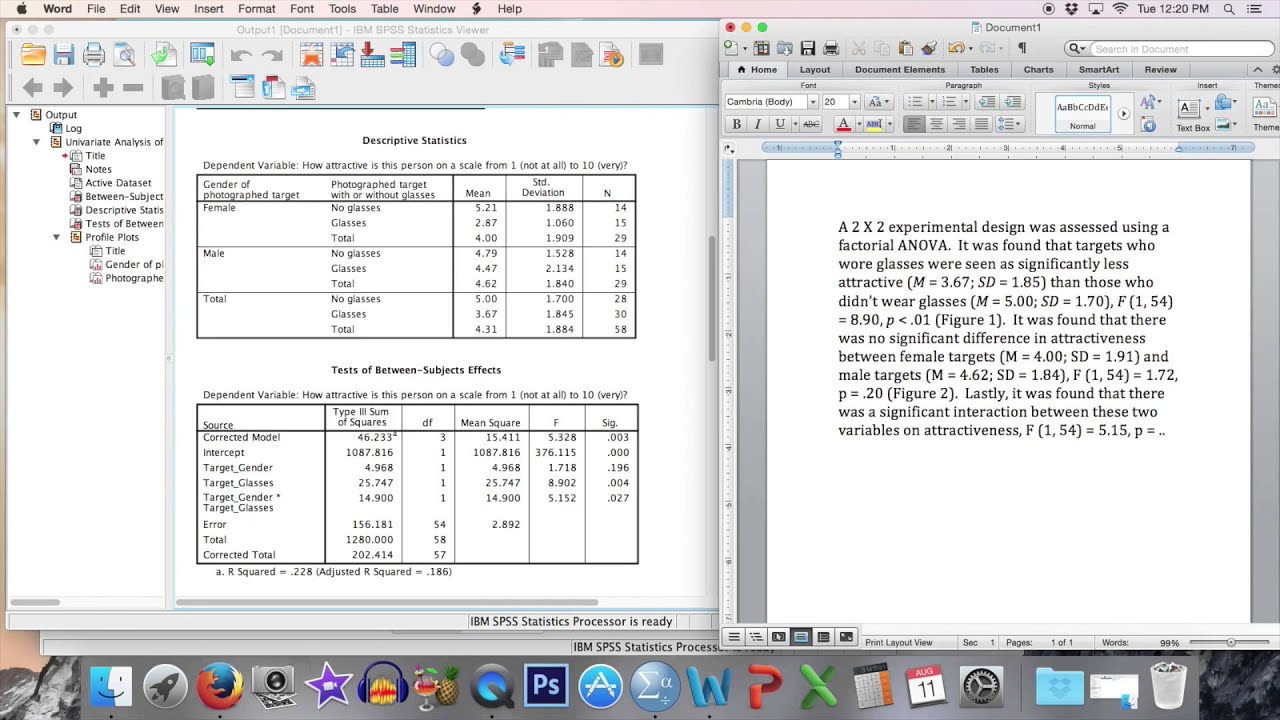
If we want to compare each group with the control group, Dunnett’s test is recommended.
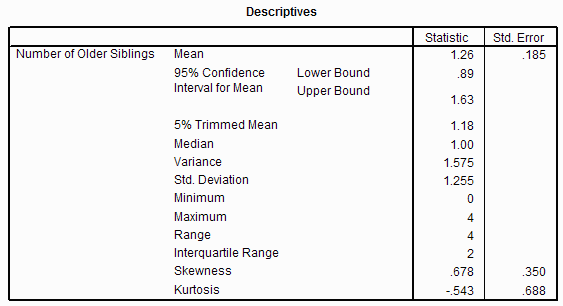
To determine which specific groups differed from each other, we further need to perform post hoc multiple comparisons. If the one-way ANOVA returns a statistically significant result, we accept the alternative hypothesis, which is that there are at least two group means that are statistically significantly different from each other. Because direct multiple use of independent samples t-test will increase the probability of type I error, one-way analysis of variance (ANOVA) is more suitable at this time. In this case, we need to compare the means among k independent samples and determine whether there are any statistically significant differences between the means of three or more independent (unrelated) groups. For example, to investigate the difference of a physiological index with different disease types, we measured the index of patients with k ( k ≥ 3) disease types. The single factor k-level ( k ≥ 3) independent sample design is a widely used experimental design method in medical experiments. In the following parts, all variables are subject to the assumption of normal distribution without special explanation. By the way, when a variable does not obey the normal distribution, it is better to report as median with its corresponding first and third quartiles (Q1–Q3) or median with its range, not as mean and standard deviation. As a result, we can conclude that the difference of mean rank has statistical significance between the experimental group and control group, which is completely contrary to the results of independent sample t-test (Table 1). After performing the test in SPSS, we have the test statistic U = 116.500 and p = 0.024. Selecting “Analyze➔Nonparametric Tests➔2 Independent Samples…” and ticking “Mann-Whitney U” in “Test Type” part. In fact, the nonparametric Wilcoxon rank sum W test is a simpler and more suitable statistical method, and the Mann-Whitney U test method should be selected in this case. Therefore, appropriate variable transformation should be performed if t-test must be used. The results show that the age in experimental group accepts the normal distribution hypothesis ( W = 0.915, p = 0.080), but the age in control group rejects the normal distribution hypothesis ( W = 0.635, p < 0.001). Selecting “Analyze➔Descriptive Statistics➔Explore…” and ticking “Normality plots with tests” in the “Plots” dialog box in SPSS. Since the sample size of two groups is less than 50, the Shapiro-Wilk test is more suitable for normal fitting test. As a result, it may be inappropriate to analyze this data by the independent sample t-test directly. Thus, the age in control group may not meet normal distribution. However, from Table 1, we can find that the standard deviation is larger than its mean value in control group. The data are quantitative data for two independent samples under single factor design.
